Between spider webs, 3D printing, geckos and AI - a week at MIT's online campus
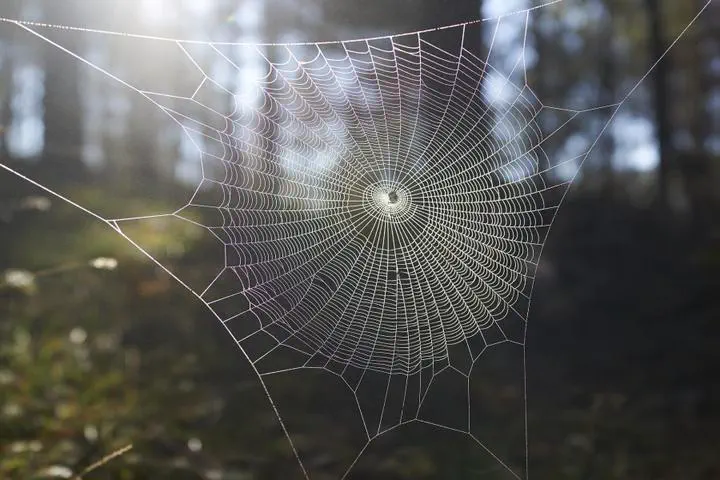
Last week I had the great opportunity to attend a short course called “Predictive Multiscale Materials Design”. The course was taught by Prof. Markus J Buehler, a Professor of Engineering and department head of Civil and Environmental Engineering at MIT. Normally held at the MIT’s campus, this year the course was online, and this made my participation possible. I would like to share with you my main takeaways from this awesome experience.
The course started giving a general overview about the topics that would be covered throughout the week. In the very first beginning, two questions were raised:
- What if atomic motions could be used to directly predict material performance?
- What if the genetic code could be used directly to predict a protein’s or biomaterial’s function?
As you can see, the concept of multiscale is taken very seriously here, covering all steps from the fundamental particles to the materials in use. In addition, something that became clear since from the beginning was the vast applicability of the techniques that were to be presented.
Prof. Buehler introduced a very interesting concept: nature creates all sorts of materials not by reinventing new building blocks every day, but by using the same constituents and “simply” rearranging them into different structures. One nice example is the Deep Sea Sponge, that is made basically of silica (glass), but, due to its macrostructural arrangement, has a very large strength. This observation would be the base for the entire course, and would drive many of the discussions.
Another idea presented was the difference between top-down and bottom-up design. The first case is more conventional and intuitive, since we’ve been using it since ever: we start with the raw material, process it in many different ways, and in the end we have a functional material with a given microstructure and atomic distribution. The bottom-up idea does exactly the opposite: it starts from the atoms, designing how they will interact, and progressively goes up in the scale, finally reaching a point where the material can be used to build whatever we need. This is fascinating, but also very challenging.
One particularly interesting example of how nature can inspire us to create innovative and better materials is the way spider webs work. Those simple structures are able to sustain considerable amount of load, even if they look really fragile. MIT has been working intensively to understand how they, again going from the characterization of the atomistic structure to the constitutive behavior and the macroscale application, and using 3D printing techniques to actually prototype new structures based on what they learn. Not to mention they have a project to create music up from the vibrations of spider webs! The two articles below give an overview about these amazing applications of science.
Here is the part where artificial intelligence (AI) jumps in. This field is already very interesting per se, but it becomes even more when applied to materials design. There are many algorithms in AI and machine learning to accomplish lots of different tasks, mainly divided into regression, classification and clustering algorithms. A relatively new algorithm called Generative Adversarial Network (GAN) was the focus of this course.
GANs have a quite specific goal: given some initial inputs (technically called training data), generate new outputs through a combination of the main features of the input space using an encoder-decoder technique. For example, given the pictures of a man and a woman, generate the picture of what could be their son. I won’t explain all the details about GANs here, since this is a rather convoluted topic, but if you are interested I invite you to read this article:
Of course, the goal of the lecture wasn’t to teach us how to generate peoples’ faces using AI, but I believe you can already anticipate was is to come. In terms of materials design, the main question to answer is: given a composite material, can we use GANs to generate a specific distribution of its basic constituents to increase its fracture energy? The answer is “yes”, and there has been a lot of research going on about this topic. In the end, what the GANs do it to capture the physical behavior of the material and to apply it to generate new (hopefully better) ones.
Still another topic was computational materials science, with a special focus on molecular dynamics. The goal is to link the atomistic and continuum perspectives, again coming back to the multiscale characteristic of the problem at hand. One example is the use of molecular dynamics, i. e., the study of how the atoms interact, to predict the Young’s modulus of a material and its fracture behavior. These atomistic simulations are visually and mechanically really close to discrete element method’s simulations.
To wrap everything up, the idea is to use molecular simulations to generate data to fill into machine learning models, enabling the design of new materials with desired properties from bottom-up. Quite fascinating! (and complex, I must say…).
One last example I want to mention is a MIT’s project inspired by geckos. The researchers studied how these tiny and under looked animals are able to get “glued” on the walls and also easily detach from them when they want to walk, using special mechanisms they have at their paws. As Prof. Buehler pointed out, the study of adhesion is very much related to fracture, since they are exactly opposites. Once more, this study went from nanostructural characterization up to the macro behavior of the geckos.
During the course, we also had the chance to work in groups to propose new ideas of projects that could be done using the technologies presented in the lectures. My group proposed the study of airplane wings that could change shape during flight according to the external conditions of wind and temperature to increase the lift-to-drag ratio, using numerical simulations and AI techniques. This proposition was inspired in the work of German researchers, and I really believe it could be something to investigate a little further. I was very fortunate, because I could interact with awesome teammates during the group’s meetings, and we had a really good time thinking about all those crazy ideas.
Finally, I can only say this was an awesome experience that I will always remember. I’m also very happy about all the people I had the chance to meet during the classes, everybody was interacting and making the lectures even more interesting. I would like to thank Prof. Buehler for sharing all this knowledge with us and for his inspiring enthusiasm about science and technology. I guess a final message could be: let’s pay more attention to nature, it probably already solved all engineering problems we could possibly think of!